Closing the gap between what companies believe and customers actually think
Why do customers become loyal to products, services, and brands? Our toolbox offers plenty of ways to find answers to this question which is the core of the market research we do every day. Tapping into a wide range of data is necessary so using the latest advances in text analytics, machine learning and statistics, SKOPOS and SEAT have developed a solution to extract insights from open questions in SEAT’s customer satisfaction programme. AI solutions provide valuable and actionable insights into what drives customer satisfaction and customer retention in the long-run.
Customer Experience (CX) is one of the key elements in ensuring customer satisfaction and loyalty. Putting customers at the centre of your business and providing them with a superior experience is an important element in distinguishing your company from its competitors. According to a Bain & Company survey, 80% of surveyed managers are convinced that they indeed provide a better customer experience than their competition. In contrast, Satmetrix surveys show that only 8% of customers agree.
This gap between companies’ internal and their customers’ outside view underlines the importance of involving customers and their feedback in the design and development of products and services. Touchpoints at which customers interact with a company need to provide an experience that is aimed at the requirements and needs of customers.
Customer experience at SEAT
SEAT recognised the need to involve customer feedback in their processes early on. The Customer Care Satisfaction Survey (CCSS) was launched with the aim of having customers monitor and audit the processes in the customer care department. But since its inception in 2010 the programme has evolved: While focusing on processes in the beginning, the survey has become more responsive and more customer-centric. Over the eight years, using the CCSS to improve their services, SEAT was able to improve their customer satisfaction by 25% across eight different markets.
When involving customer feedback in your service design, different expectations and perceptions play a critical role. Customers do not think of themselves only as customers and understanding their needs, seeing the world with their eyes, is crucial to providing the right services at the right time.
Focus groups and face-to-face interviews can offer this perspective on a small scale. Scaling the generation of such insights to a global dimension requires innovative solutions. SEAT, for example, collects feedback in eight markets and in nine different languages – an amount and complexity that is difficult to handle manually. While online customer surveys often have invited customers to provide open feedback, it is rarely analysed with a clear research question in mind. The latest developments in machine learning and text analytics research, however, allows us to analyse large amounts of text data and investigate it in relation to customers’ expectations and their satisfaction with the services and products.
Together with SEAT, SKOPOS developed an AI solution based on those developments to extract aspects of SEAT’s customer care process and identify those aspects that are most important to customers. By improving these aspects in particular, SEAT gains a leverage to increase both their customer satisfaction and retention.
Under the hood
While AI is one of the biggest future trends in nearly every industry, it is also a very generic word used for a wide range of applications so it’s useful to take a look at what is actually going on under the hood. Text analytics solutions face several problems with human-written text, for example: ambiguous words, irony and sarcasm or typos. Pre-processing of the texts and involving external knowledge is a necessary first step.
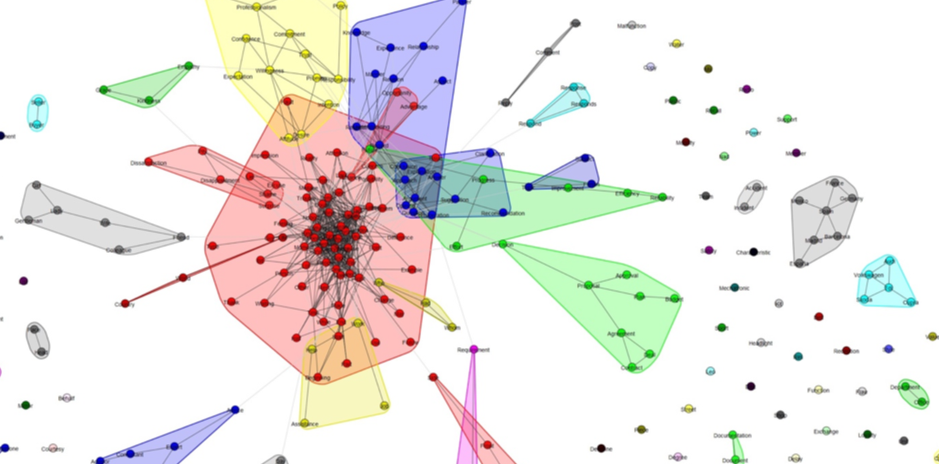
The first step is to extract what customers are writing about, i.e. the aspects of the service experience they give feedback on. After translating all responses to English, a language model is used to identify relevant keywords (e.g. “car”, “vehicle”, “workshop”, “mechanic”, “person” etc.). As most language models perform best in English, it is the most useful language to work with. Further using models encoding relationships between words (word-embeddings), we aggregate the keywords to aspects. This recognises that “car” and “vehicle” are referring to the same concept and therefore should be considered together in the analysis.
As we are interested in how customers are writing about their experience, using state-of-the-art sentiment analysis libraries, we extracted the sentiment for each response relating to a specific aspect.
While many solutions finish at this point and provide a colourful word cloud, we aimed to go a step further by relating the data from open responses to the overall customer satisfaction rating. Thereby, we are able to show not only what has been said often but also highlight aspects that are particularly relevant to customers’ satisfaction. This provides actionable insights and shows SEAT what leverage they have to further improve customer satisfaction.
Conclusions
Using data from eight countries over several years, many different insights are available on different aspects of the customer experience. One result stands out that underlines the benefit of our machine learning-based solution: While customers often talk about their car and why they have been in touch with SEAT’s customer care (i.e. it would be large and visible in your traditional word cloud), it is actually the experience at the touchpoint itself, that has the strongest relationship with overall satisfaction. The more positively customers wrote about the person they had spoken to, the more satisfied they were. Additionally, a good-will policy is a valuable tool for SEAT with measurable impact.
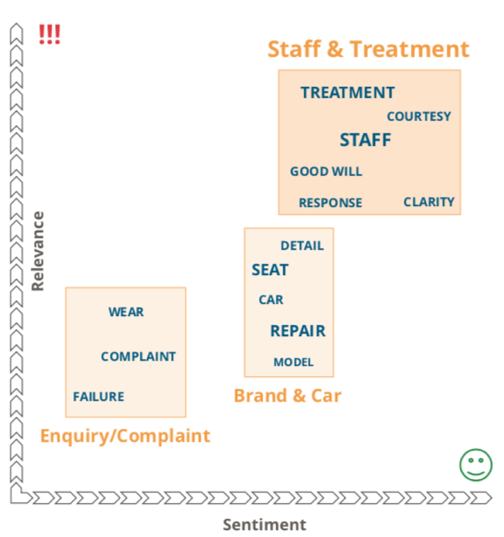
It might seem obvious, but having the data actually show this relationship in a consistent manner across the customer sample, is important to derive recommendations on what changes to processes or tools can be made.
To answer a research question, it is necessary to collect data relevant to the question. The case study summarised here only involved data from a single touchpoint: The customer care satisfaction survey. For the central question on how to have satisfied and loyal customers, additional data is desirable. If data were available from all touchpoints along the customer journey, data analytics could provide even deeper insights on how customers perceive and react to their individual customer experience. What are critical events and what is driving customer satisfaction along the customer journey? What influences customer retention? What is the economic impact of marketing activities on customer retention?
These questions are relevant to nearly any business. Data necessary to answer these questions, however, is rarely available. Companies working on implementing a data strategy are on course to allow analytics provide them with answers making efficient decisions on strategy, marketing, sales and human resources possible. Companies failing to make use of data and the capabilities of AI solutions, will find themselves too blind to bridge the gap between their inside view and the outside view of their customers and the Delivery Gap will only become larger for them.
Data science and data analytics will fuel customer experience research as the amount and complexity of available data will further increase. Integrating data from multiple sources, combining them into a coherent analytical framework, and deriving actionable insights is the next step for state-of-the-art CX research. Further potential lies in using those results from CX research and the resulting models for personalising the customer’s individual customer journey to their needs and requirements. Data science provides solutions for both ends: the CX research perspective and the operational perspective in giving recommendations e.g. to customer care representatives when talking to the customer.
The case study shows the potential of using AI in the context of customer experience research. It was only a first step in the long journey of implementing AI in real-world applications. In our experience, small projects with a clear research question and some initial data can help to spark new ideas of how strategic questions, data, and the right toolset can come together to provide actionable insights in what customers think, do and feel.
Further details are available in the papers published from the ESOMAR Fusion 2018 conference www.esomar.org.