As marketers, we are aware of the frequent changes in the customer behaviour. Customers’ motivations, expectations, purchasing power and their habits continuously go through rapid changes. Even life events have become more difficult to predict than those in the past. Marketers who fail to predict these shifts, and fail to build strategies for new realities and lives of their customers, struggle to compete in the fast-changing world of today. Winning over ever-evolving customers require deep holistic insight and a willingness to design exceptional, personalized customer experiences at every touchpoint in the customer journey.
This article discusses harnessing Omnichannel Customer Feedback data using Deep Learning and integrating with Net Promoter Score (NPS) Survey Data to deliver superior CX. We will also show how we’ve applied those techniques for our client, Telco, in Indonesia.
Setting the context: delivery gap in customer experience
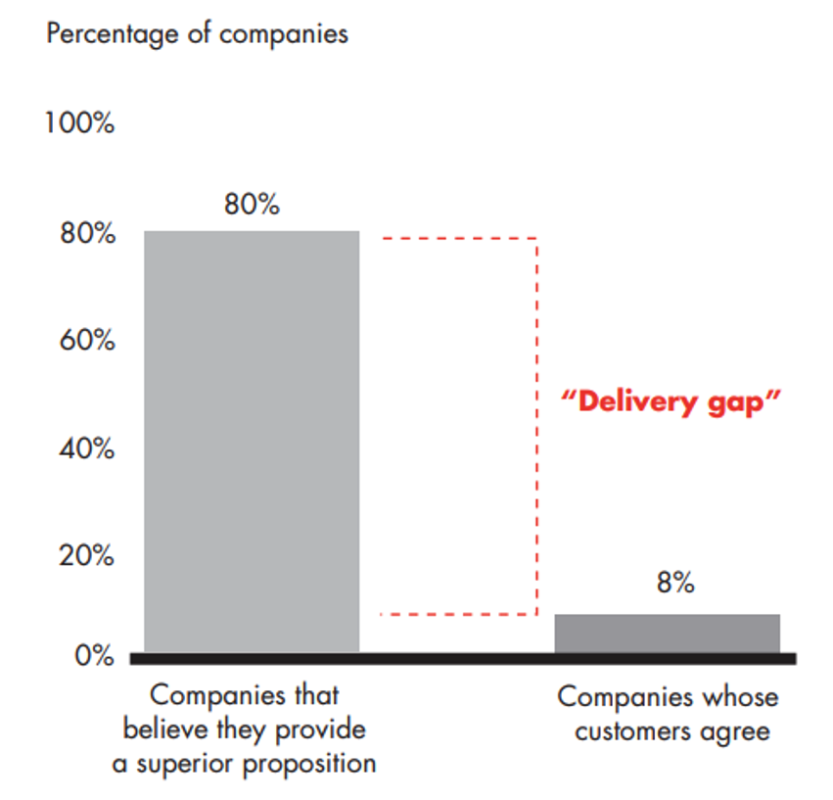
Source: Bain Customer-Led growth diagnostic questionnaire, n = 362;
Satmetrix Net promoter database, n = 375
There is a wide delivery gap between customer expectations and brand delivery. Interestingly, brands are unaware of this vast delivery gap, and they have a perception of delivering superior customer experience.
Customers of today expect more from brands, and they do so because of the existence of brands that are delivering exceptional experiences.
Therefore, when certain brands fail to keep up, customers take notice and they form a negative perception about those brands.
The ideal way of measuring the customer experience
The CXM (Customer Experience Management) tool should do three things for organizations to guide them to growth-oriented decisions – LISTEN, THINK and ACT.
- Listening involves the collection of customer feedback at multiple channels in a customer journey. These customer interactions can be on multiple platforms like website, in-app, chatbots, in-store etc.
- Thinking part is crucial. The tool we used in this thesis helps us to discover the top concerns and improvements to deliver data-driven customer experience. Our tool uses Deep Learning technologies in analysing customer textual feedback. It automatically categorizes Customer Feedback into meaningful insights.
- Taking instant actions in the right direction is what a CXM can do for various organizations. The right tool should be something that could give organizations instant insights and coax the action by the relevant department and close the feedback loop by turning customer complaints into customer success.
The CXM tool we are experimenting with, promises us these aspects of customer experience and enables us to harness textual data from chatbot in improving experience for our Telco client in Indonesia.
Client’s key challenges
Our client was using a chatbot as a first point of contact with the consumers. If chatbot could not address the consumer’s concern, it would pass the conversation to human agents. However, even when utilising the chatbot, the client was still facing following issues:
- Satisfaction for chatbot was very low, with average ratings of 2.7.
- While the goal of the chatbot was to bring efficiency and reduce human conversations, more than 91% of the conversations were forwarded to human agent, validating inefficiency of the chatbot.
- Churn rate increased significantly after deployment of chatbot.
How our CXM tool helped?
Prediction of Topics and Subtopics from Bahasa Indonesia Chatbot Conversations
Our CXM tool could read Bahasa Indonesia text, and it extracted key topics for our client. It also predicted top subtopics within each big topic.
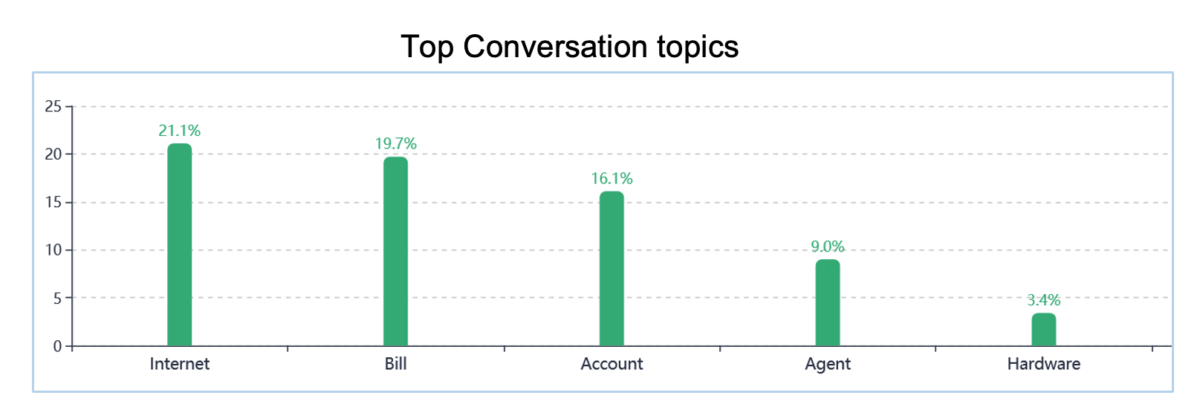
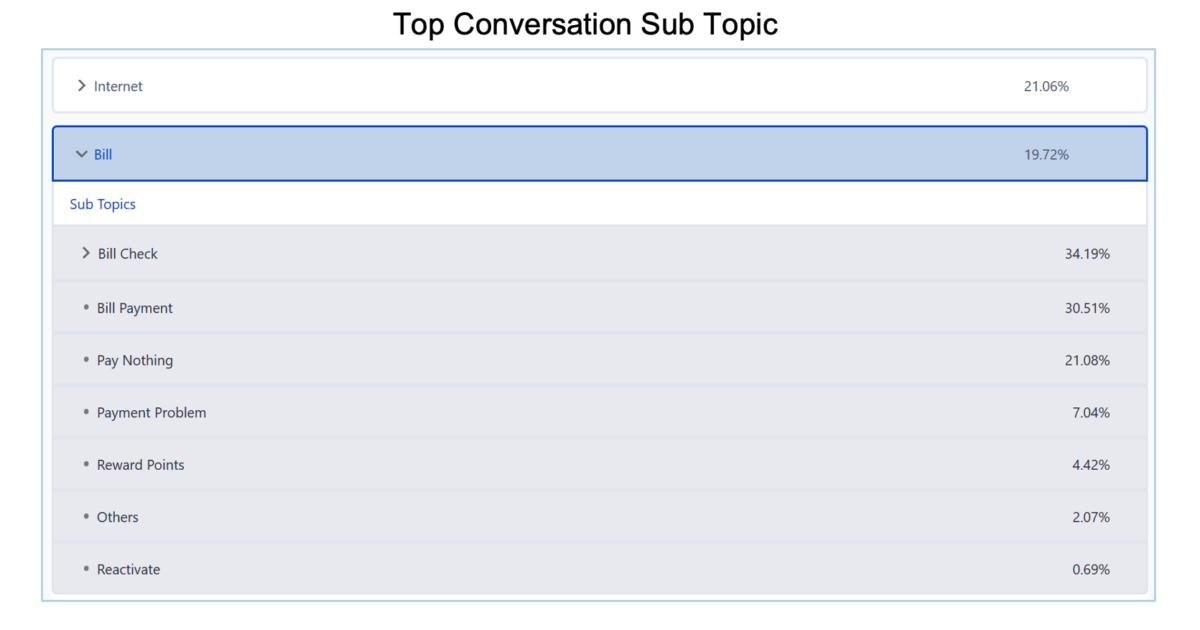
- Our Natural Language Processing (NLP) Algo analyzed conversations and came up with 12 major topics and 86 subtopics.
- Internet, bill and account were the three top themes emerging from NLP analysis.
- The top subtopics, where the consumer was conversing with the bot, were distributed across major topics. ‘internet not working’, ‘agent request’, ‘Account check’, ‘bill check’ and ‘bill payment’ and ‘pay nothing’ request made up around 50% of conversation volume.
Priority Prediction:
Our CXM tool predicted priority of conversations ranging from very urgent to irrelevant. It helped the client with prioritizing and addressing most urgent conversations. This prediction was done on the individual conversation level & the topic level.
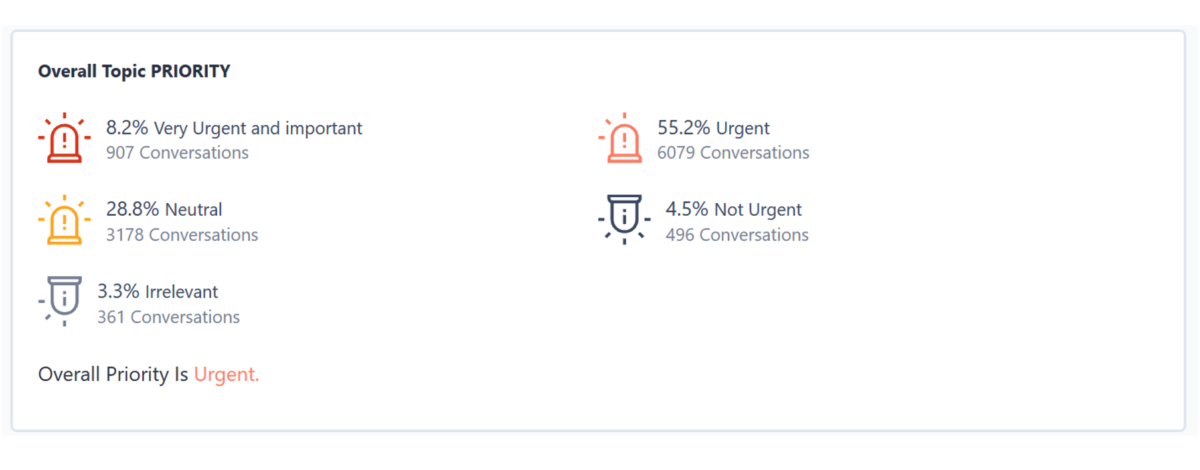
Sentiment Prediction:
Along with priorities, our CXM tool also predicted sentiment related to each topic, subtopic and conversation.
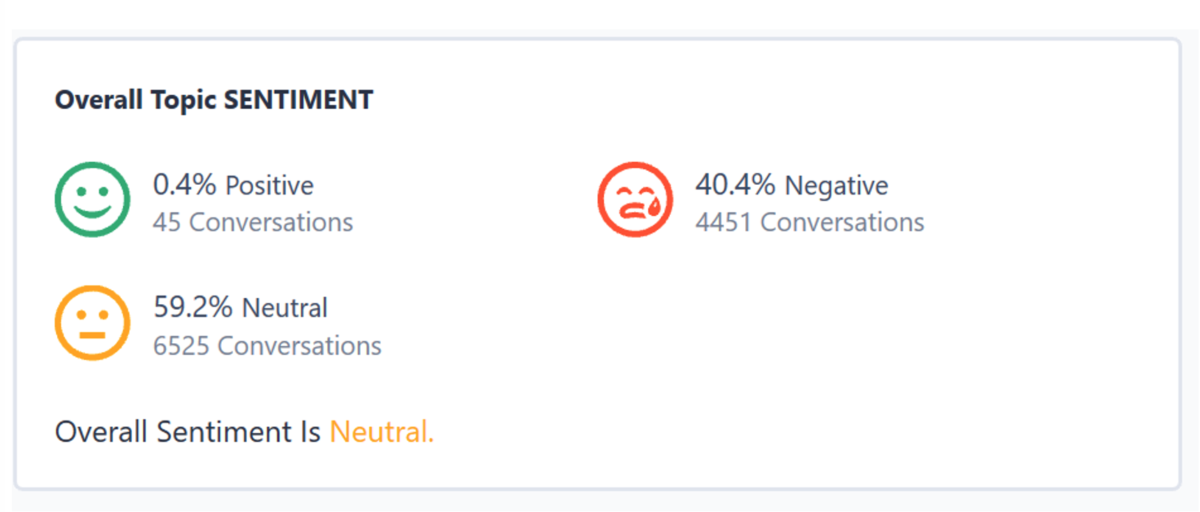
- Internet, line, coverage and account were the highest priority topic across all conversations.
- At the subtopic level data, we see account termination, phone line not working, internet speed and internet not working as having much higher priority over the other topics.
- Majority of the negative sentiments were coming from internet, account, bill and hardware.
- Most conversations were negative due to complaints of ‘internet not working’ and ‘internet speed’ issues where customers complained about internet being disconnected and about lower internet speed issue respectively.
Intent Prediction:
Our NLP model also predicted the intent of each conversation so that the customer success can have a better priority and understanding of which conversations to access first.
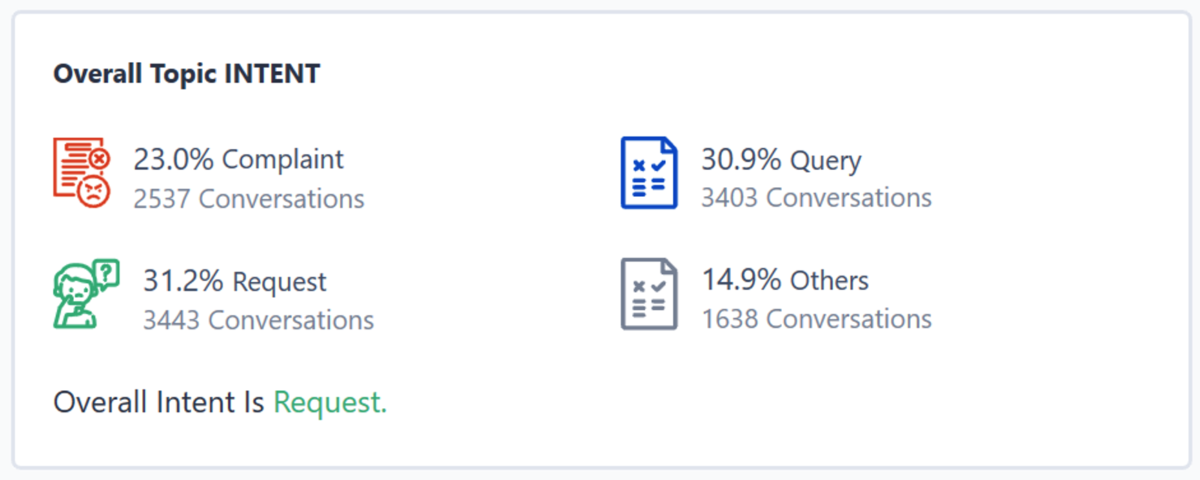
How did the output of our Intelligent CXM tool help in reshaping CX for the client?
- Redesigning UX:
- Our client used the topic and subtopic classification to redesign UX of the chatbot. Instead of entering their query to the chatbot, the customer was allowed a choice of topics and subtopics so that their queries can be entered faster and addressed better.
- FAQs, documentation and help articles were provided to facilitate the interaction with the bot, and minimise human interaction.
- Ticketing for Unresolved conversations: Our CXM tool helped the client in identifying unresolved conversations and creating an automatic alert for customers close to churn out.
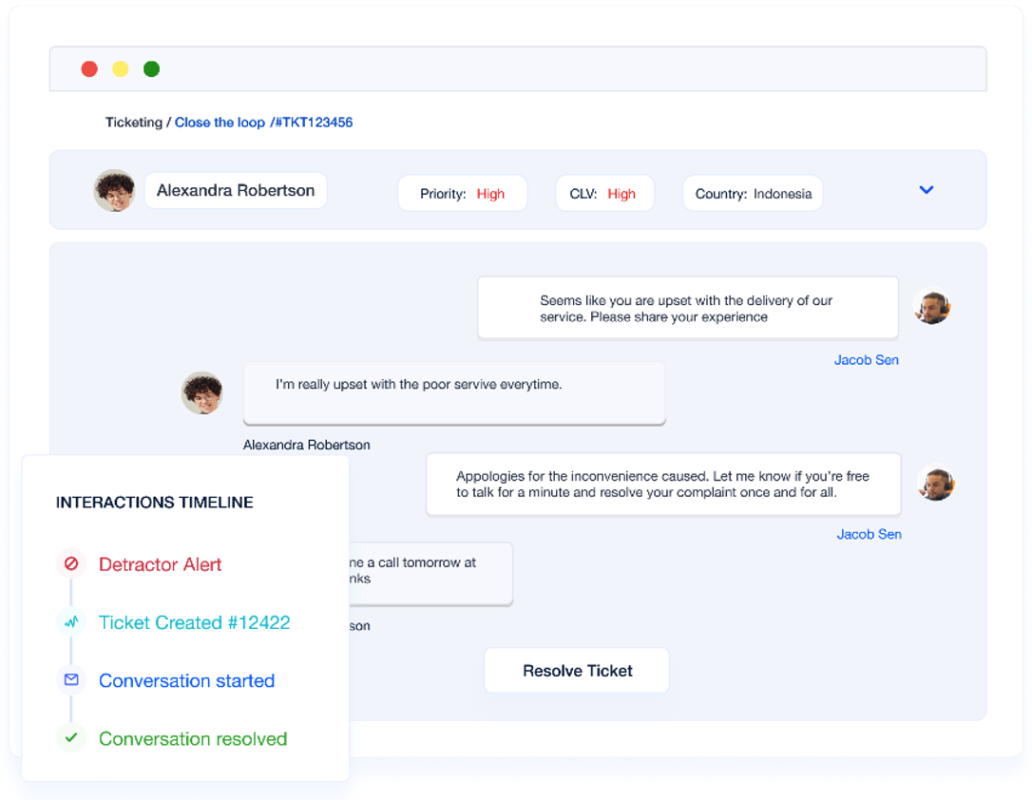
Key results:
- Chatbot satisfaction improved from 2.7 to 4.1 in 2 months.
- 54% of the conversations with chatbot ended satisfactorily and decreased the metric of 91% human agent interaction requests.
- Churn rate was reduced by 15% in 2 months.
As part of our research, we would like to lay down key ground principles for measuring and delivering superior customer experience:
- Delivering superior CX requires deep listening, thinking and actions. Brands need to move from legacy CX measurement methods to agile tools which can measure omnichannel feedback and provide proactive actions for the client.
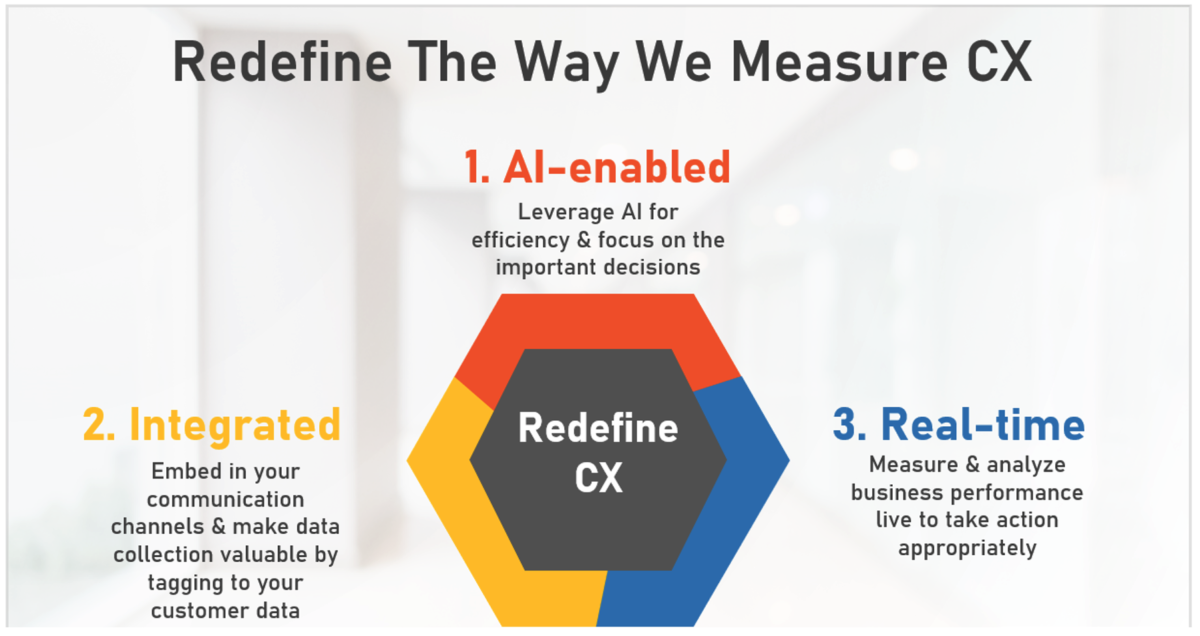
- Deep Learning based Text Classification techniques show high accuracy and efficiency in analysing textual feedback in local language such as Bahasa Indonesia.
- Marketers of today need to monitor individual conversations through intelligent AI-based systems, predict churn out and take proactive actions.
- Finally, brands need to focus on good profits over bad profits. Our work with Telco showed longevity of good profits. While the actions suggested by us, such as network upgrade in key areas, were expensive in the short term, the benefits delivered by those actions produced a big jump in brand loyal customers and led to revenue uplifts.