Without question, automation and the rise of AI based MR-specific applications have driven process improvements, time savings and cost reductions across a host of MR functions. We know this because automation is nothing new, its impact measured and proven over many years.
But it’s also true that it hasn’t transformed our jobs or businesses beyond recognition. That’s because MR’s technology evolution has been a gradual process, and the uptake of new tools and techniques – aside from early adopters – has taken a measured path.
A well-trodden path
Our industry is no different to others when it comes to the lifecycle of new products and solutions. Usually, they follow the standard route from development through to decline, as illustrated below.

In MR, technology has evolved on a continuous basis, with a particular focus on automating more of the process-based activities we perform.
However, what has happened more quickly in the past few years is the level of capability that automation delivers – moving from simple, process-based work to deeper, more complex and more cognitive aspects of the research lifecycle. And this is where commentators see both a risk and an opportunity: the threat of human redundancy, but the chance to deliver more, at lower cost, in less time.
Predictive analytics, intelligent automated creation of surveys from Word documents and action modelling are just a few examples of the step-change that we’re now seeing. Based on the leaps forward we’ve made in AI, these types of solutions are slowly creating a new ‘normal’ for MR practitioners.
The roots of AI
The term ‘artificial intelligence’ was coined by John McCarthy in 1956 when he held the first academic conference on the subject. The main advances over the past 60 years have been in search algorithms, machine learning algorithms and integrating statistical analysis to allow us to understand the world at large.
AI’s entry into MR has also been a methodological one, with an extended incubation period from the 1970s to the early 2000s. In terms of actual AI products, we’ve really only seen them emerge in the last five to 10 years – with varying degrees of readiness for MR professionals.
If we map this onto our product development lifecycle, it becomes clear how far we still have to go before we reach true maturity. Or is that all about to change?
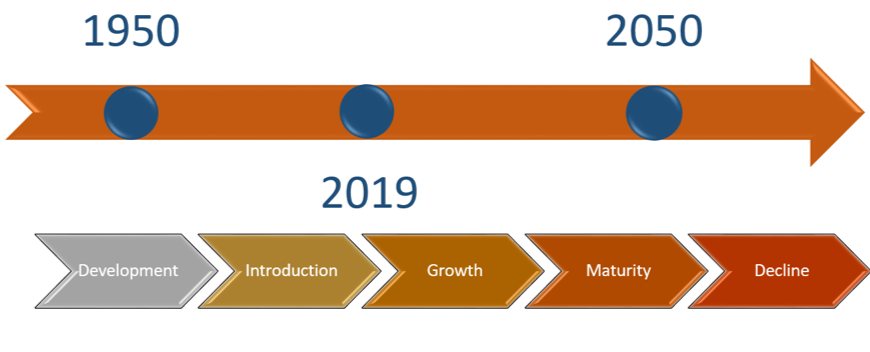
Growing clever
With continuing pressure to increase efficiency and speed, we’re now seeing an accelerated introduction of new AI solutions that delve much deeper into the research process.
This is generating greater clarity about the benefits that more sophisticated tools can deliver – allowing agencies to recognise that they can exploit AI beyond operational and process efficiencies and apply it to research product development, analytics and client delivery.
This is driving exponential growth in the implementation of AI tools across all aspects of MR, most notably in the areas of predictive analytics, social media analytics and action management.
In these areas, the latest AI techniques such as deep learning neural networks and Natural Language Processing (NLP) are harnessed to automatically uncover insights, traditionally a task that relies on human interpretation of analytics data.
For example, tools now exist to automatically uncover concepts hidden within reams of text data, unlike standard text analytics tools which still require businesses to know what issues or trends they’re looking for.
By moving beyond a model of analysing research data based on pre-defined category models, organisations can continually learn with an ‘automated eye’ on what’s changing in their world. Even in their early adoption, such tools deliver accuracy results that often exceed 90% and outperform human analytical capabilities.
Machines can learn too
These numbers have been proven in applications within and beyond MR. What’s more, faster and increasingly powerful AI techniques mean accuracy is only getting better.
Put in context, let’s look at the famous example of chess playing. Over 20 years ago, IBM’s Deep Blue beat Garry Kasparov under standard chess tournament rules. Even at that time, Deep Blue was capable of searching through hundreds of millions of move possibilities per second, much faster than any human brain.
In 2015, Matthew Lai developed a hugely advanced chess engine, Giraffe, which moved beyond sequential processing and instead simulated intuition, learning how to beat opponents by playing itself. It could do this through the power of deep neural networks. And while Giraffe was discontinued as Lai moved to Google DeepMind, the leap in capabilities that it demonstrated serves as a reminder of the level of potential that AI technologies offer for performing deep, learning-based, cognitive MR work.
AI will always need humans
A scary scenario for all of us who work in MR? Looking at accuracy rates and the raw capabilities of AI tools alone, it’s easy to assume that we don’t need people to deliver better, deeper insight to our clients. This is an all-too-common misconception. Embracing AI tools to do some of our deeper learning means that we have to elevate our own roles to deliver better, more accurate guidance and decision-making.
That’s because AI is a catalyst for, not a replacement of, human knowledge and understanding. It doesn’t deliver all the answers fully-formed but instead provides the most accurate and appropriate insight to guide humans to the answers they need.
AI is a catalyst for, not a replacement of, human knowledge and understanding. It doesn’t deliver all the answers fully-formed but instead provides the most accurate and appropriate insight to guide humans to the answers they need.
Indeed, one of the key benefits of automation is the increased need for skilled people. That’s where organisations need to define best practice approaches in terms of AI and human roles. If an increasing number of cognitive tasks are capably handled by automation, research teams must evolve into specialist insight hubs, where researchers become data scientists and reports offer strategic business guidance.
Where next?
In the field of AI, expectations always seem to outpace the reality. Despite our friends in the chess world and after decades of research, no computer has come close to passing the Turing Test, a model for measuring ‘intelligence’. This suggests that we are some way from the maturity stage, although some, including Forrester, predict that many people expect that AI will have a noticeable impact on their life within five years..
As we see increasing development spend focused in this area in other sectors – facial recognition solutions for ecommerce, translation and voice recognition solutions for business, and imaging and diagnostic solutions in the medical field, for example – we need to understand what this means for our own industry.
In our view, MR won’t be significantly disrupted by AI in the years ahead, but we are harnessing the possibilities for transformation that it can deliver to key aspects of our work.
And we do know there will be an increasing number of niche AI solutions in MR. Even though these won’t attract mass adoption in the immediate future, we need to be ready to embrace all of the potential they offer – as well as being armed for the pitfalls that we’ll encounter as we merge our human skills with our increasingly cognitive AI toolkit.